Do not Simply Sit There! Start YouTube Subscriber Increase Service
작성자 정보
- Lester 작성
- 작성일
본문
The YouTube formula, an intricate system created to advise video clips to customers, has seen significant innovations because its creation. These formulas are critical to user interaction, content discovery, and system development.
To appreciate the upcoming innovations, it's important to understand the existing state of Youtube Marketing Agency's recommendation system. Historically, YouTube made use of basic metrics such as sights, suches as, and shares to recommend web content. In time, the algorithm progressed to incorporate more advanced signals, including watch time, individual interaction, and customized watching habits. The introduction of deep understanding methods further enhanced the formula's capability to anticipate individual preferences and advise pertinent content.
Today, the YouTube algorithm operates on a multi-layered framework. The first layer identifies a pool of prospective videos a user could such as, while the second layer ranks these videos based upon many variables, including user background, engagement metrics, and contextual signals. The performance of this system is undeniable, yet it still deals with difficulties such as filter bubbles, material diversity, and the need for real-time adaptation to altering user choices.
By analyzing user communications as they happen, the algorithm can refine its forecasts and supply more accurate material suggestions. An individual may like educational web content during weekdays and amusement on weekend breaks. Adaptive learning systems overcome this by making use of strategies such as federated knowing, which enables the formula to learn from decentralized information sources without jeopardizing customer personal privacy.
: One of the objections of existing suggestion systems is the production of filter bubbles, where customers are repeatedly revealed comparable web content, limiting their exposure to diverse point of views.: The ability to adjust in real-time ensures that the formula stays relevant also as user choices progress. By identifying arising trends and individual rate of interests in real-time, the algorithm can promote new and appropriate web content more effectively.
To illustrate the practical effect of flexible knowing systems, take into consideration a hypothetical instance research study where YouTube implements this technology.
Situation: YouTube presents flexible knowing systems in a phased rollout, beginning with a subset of individuals in a specific region. The objective is to examine the influence on individual engagement, content variety, and general contentment.
Approach: The rollout includes integrating real-time feedback loopholes, context-aware recommendations, and multi-modal data combination into the existing algorithm. User communications, enjoy times, and feedback are checked continually to gauge the system's efficiency.
Results:.
While the advantages of adaptive knowing systems are clear, their application is not without difficulties.: Leveraging real-time user information elevates privacy concerns.: Guaranteeing that the algorithm remains objective and fair is a continuous obstacle.
Future instructions for research study and development consist of discovering much more innovative AI strategies, such as reinforcement discovering, to even more boost the flexibility and efficiency of referral systems. Additionally, incorporating customer comments more perfectly into the algorithm's understanding procedure can supply beneficial understandings and boost its efficiency.
The advent of adaptive knowing systems marks a considerable leap in YouTube formula optimization, using a more dynamic, context-aware, and individualized technique to content referral. By addressing the constraints of existing algorithms and leveraging the most up to date innovations in AI and ML, these systems assure to improve customer interaction, material variety, and general fulfillment. As YouTube continues to advance, the assimilation of adaptive discovering systems will definitely play a crucial duty fit the future of content discovery and suggestion.
These formulas are essential to user involvement, web content exploration, and platform development. The introduction of deep knowing techniques even more enhanced the formula's capacity to forecast individual choices and suggest pertinent material.
The first layer determines a pool of prospective videos a customer may like, while the second layer ranks these video clips based on numerous aspects, including user background, interaction metrics, and contextual signals. By recognizing emerging fads and individual rate of interests in real-time, the algorithm can advertise new and relevant web content more properly. By attending to the limitations of current formulas and leveraging the most current improvements in AI and ML, these systems assure to enhance customer engagement, material diversity, and general fulfillment.
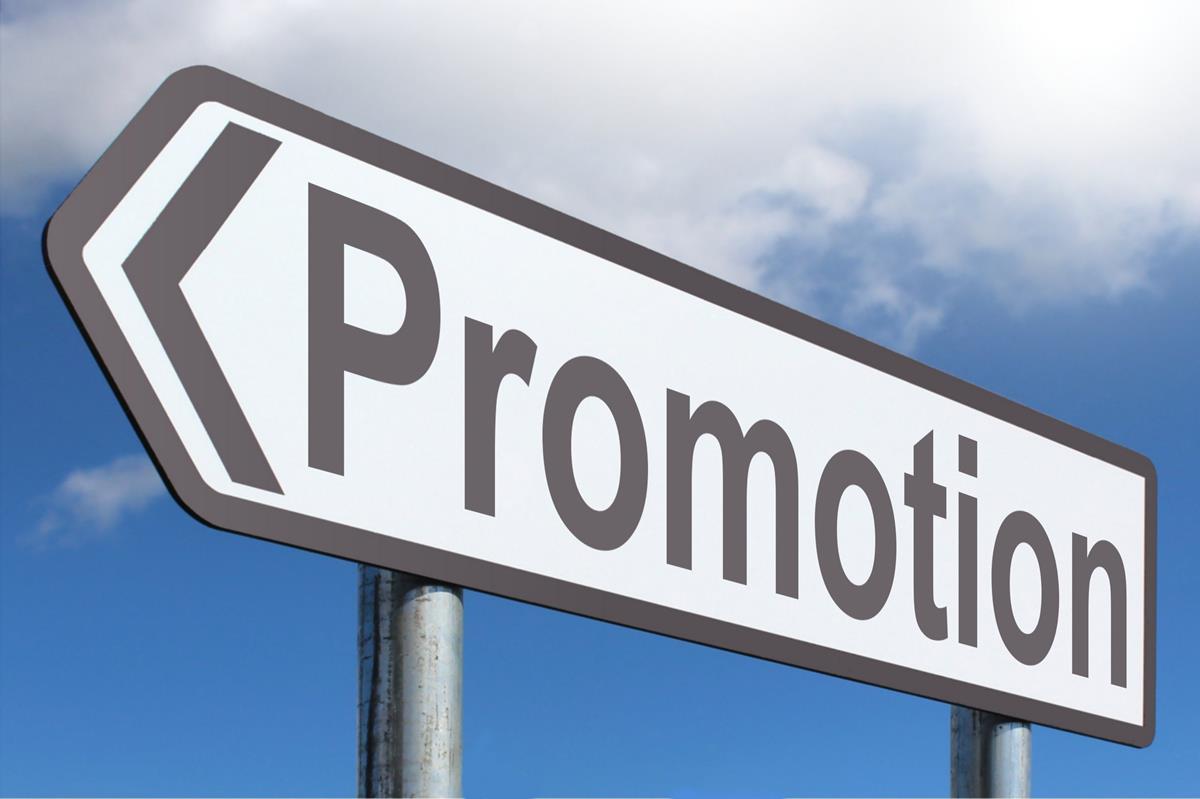

The Next Jump: Adaptive Understanding Equipments
By analyzing user communications as they happen, the algorithm can refine its forecasts and supply more accurate material suggestions. An individual may like educational web content during weekdays and amusement on weekend breaks. Adaptive learning systems overcome this by making use of strategies such as federated knowing, which enables the formula to learn from decentralized information sources without jeopardizing customer personal privacy.
: One of the objections of existing suggestion systems is the production of filter bubbles, where customers are repeatedly revealed comparable web content, limiting their exposure to diverse point of views.: The ability to adjust in real-time ensures that the formula stays relevant also as user choices progress. By identifying arising trends and individual rate of interests in real-time, the algorithm can promote new and appropriate web content more effectively.
To illustrate the practical effect of flexible knowing systems, take into consideration a hypothetical instance research study where YouTube implements this technology.
Situation: YouTube presents flexible knowing systems in a phased rollout, beginning with a subset of individuals in a specific region. The objective is to examine the influence on individual engagement, content variety, and general contentment.
Approach: The rollout includes integrating real-time feedback loopholes, context-aware recommendations, and multi-modal data combination into the existing algorithm. User communications, enjoy times, and feedback are checked continually to gauge the system's efficiency.
Results:.
- Customer Interaction: Within the first three months, customers in the examination group show a 20% boost in average watch time and a 15% surge in communication prices (sorts, comments, shares) compared to the control group.
- Web content Variety: Analysis discloses a 25% rise in the diversity of web content consumed by users in the test group. Customers are revealed to a more comprehensive series of genres and designers, decreasing the occurrence of filter bubbles.
- Individual Fulfillment: Studies show a 30% enhancement in individual fulfillment scores, with users reporting that the suggestions really feel a lot more appropriate and individualized.
- Web content Discovery: New and emerging material makers experience a 40% rise in exposure and involvement, as the algorithm better advertises fresh and relevant material.
Challenges and Future Instructions
While the advantages of adaptive knowing systems are clear, their application is not without difficulties.: Leveraging real-time user information elevates privacy concerns.: Guaranteeing that the algorithm remains objective and fair is a continuous obstacle.
Future instructions for research study and development consist of discovering much more innovative AI strategies, such as reinforcement discovering, to even more boost the flexibility and efficiency of referral systems. Additionally, incorporating customer comments more perfectly into the algorithm's understanding procedure can supply beneficial understandings and boost its efficiency.
Final thought
The advent of adaptive knowing systems marks a considerable leap in YouTube formula optimization, using a more dynamic, context-aware, and individualized technique to content referral. By addressing the constraints of existing algorithms and leveraging the most up to date innovations in AI and ML, these systems assure to improve customer interaction, material variety, and general fulfillment. As YouTube continues to advance, the assimilation of adaptive discovering systems will definitely play a crucial duty fit the future of content discovery and suggestion.
These formulas are essential to user involvement, web content exploration, and platform development. The introduction of deep knowing techniques even more enhanced the formula's capacity to forecast individual choices and suggest pertinent material.
The first layer determines a pool of prospective videos a customer may like, while the second layer ranks these video clips based on numerous aspects, including user background, interaction metrics, and contextual signals. By recognizing emerging fads and individual rate of interests in real-time, the algorithm can advertise new and relevant web content more properly. By attending to the limitations of current formulas and leveraging the most current improvements in AI and ML, these systems assure to enhance customer engagement, material diversity, and general fulfillment.
관련자료
-
이전
-
다음
댓글 0개
등록된 댓글이 없습니다.